A recent report by the Global Market Insights reveals that the market of artificial intelligence applied to health care in 2016 reached 750 million dollars and it is expected to grow by 2024 with an annual growth rate of 40% per year.
The field of artificial intelligence can be represented through a Venn diagram that highlights the conceptual relationships between the different techniques.
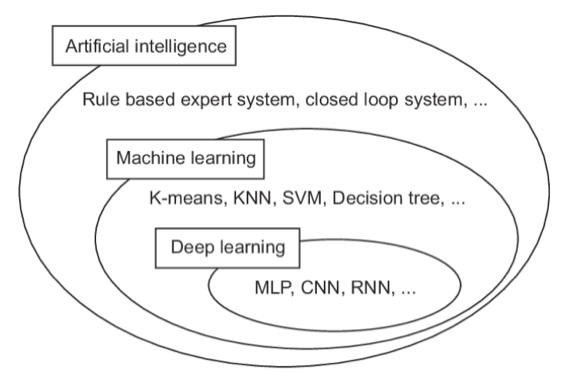
From the diagram it is possible to see that deep learning is a special case of representation learning, which in turn is a subset of machine learning and the whole is a sub-division of artificial intelligence technologies. The term machine learning refers to a set of techniques and approaches aimed at solving certain types of problems that require the algorithm to learn from a certain set of data. The study of machine learning algorithms is currently a very active field of research, which has registered many successes in the field of image recognition and understanding of spoken language. In these areas, in fact, there are very difficult problems to solve with “hard coded” programmes, whose functioning does not involve training. In this approach, the programmes subjected to training learn a hierarchy of data concepts (known as data representation). When we learn representations from other representations, we talk about a profound stratification of learning: deep learning.
APPLICATIONS OF NEURAL NETWORKS IN MEDICAL IMAGING
In the health field, artificial intelligence is widely used in medical imaging, as it allows a better characterization and a faster identification of metastases that can improve the outcomes of therapies. In particular, deep learning techniques are mostly used for those aspects related to medical imaging, which will be analysed below.
CLASSIFICATION OF EXAMS AND IMAGES
One of the first uses of deep learning algorithms in medical imaging is the classification of images. Usually there is a certain number of images as inputs and a single diagnostic variable in the output (presence / absence of the disease). As for datasets, they are much more restricted in medical imaging than computer vision in general (hundreds / thousands of samples against millions). For this reason, the transfer learning technique is often used, which basically consists in pre-training the neural network to circumvent the data training needs of the programme. There are two mostly used transfer learning strategies:
- Use a pre-trained network as features extractor;
- Recalibrate (perform a "fine tuning") a pre-trained medical data programme.
Both strategies have been used successfully in many cases, although the latter is proving better.
CLASSIFICATION OF AN INJURY OR AN OBJECT
The classification of an injury means to classify a small part of the medical image, like a cancer in a specific organ. To this end, the information about the local injury and overall information on the location of the injury is important. In general, generic deep learning algorithms are not suitable for this type of activity, so an approach used in many cases is the multi-stream one. Some studies have used concatenated multi-stream networks and a combination of convolutional and recurrent networks, the use of recurrent components helps to make the analysis independent of the image size.
In the case of 3D image analysis, instead, the networks must be readjusted. In addition to convolutional neural networks, other types of architecture have also been used for the purpose, including RBM (Restricted Boltzmann Machine), SAEs (Stacked Autoencoders) and SAE (Convolutional Stacked Autoencoders). Unlike the convolutional neural networks, the last mentioned solution is characterized by an unsupervised pre-training with scattered autoencoders. Another interesting approach is multiple instance learning (MIL) combined with deep learning methods. This procedure achieves excellent results compared to the extraction of features by hand.
DETECTION OF ORGANS AND REGIONS
In the pre-process of the analysis of medical images the anatomical detection of objects is a very important step. In the detection of 2D objects, the most used architectures are convolutional networks, given their good results. It seems that even the RNNs can achieve excellent results in this activity.
INJURIES DETECTION
One of the crucial problems for diagnosis is the injuries detection in medical images or in identifying them in the image. Also in this case, the great majority of studies carried out so far used convolutional neural networks for analysis by pixel or voxel. There are some technical differences in the detection and classification of injuries with neural networks and, apart from these, the detection and classification are solved in a rather similar way.
SEGMENTATION OF ORGANS OR SUBSTRUCTURES
Organ segmentation is an important part of automatic diagnostic systems and allows quantitative analysis of the volume and shape of the regions of interest. This activity consists in identifying the pixels or voxels that form the outline, volume or surface of the desired object. Most of the articles on the use of deep learning algorithms applied to medical imaging deal with segmentation. For this activity, many architectures based on convolutional network and RNN have been implemented. The best known of the convolutional networks implemented for segmentation is the U-Net neural network. In addition to convolutional networks, RNNs networks have also been used recently for segmentation.
INJURIES SEGMENTATION
Injury segmentation is a mixed approach between object detection and segmentation. For such activity, U-Net and similar architectures are used to have a global and local analysis of the image.
IMAGES RECORDING PROCESS
The recording of medical images consists of calculating a transformation of coordinates from one medical image to another. The most commonly used methods are:
- Use deep learning networks to estimate a similarity measurement between two images for an iterative optimization;
- Directly predict the transformation parameters with regression models.
Even in this case the most frequently used architectures are convolutional. For the images recording activity different architectures are used, including SAE and CNN. However, it is still not clear which method is the best.
CONCLUSIONS
Recent studies have demonstrated the effectiveness of different deep learning algorithms applied in automatic diagnostic systems. In the future, further performance improvements and an increasingly widespread and effective use is envisaged. Thanks to artificial intelligence, it is possible to automate the detection, monitoring, therapy prediction and therapy response.
For further information visit our website.