Deep learning (DL) is a branch of machine learning and one of the most promising technologies in the field of artificial intelligence (AI), but how does it differ from machine learning (ML) and traditional AI?
In this article, we will try to understand what deep learning is and how it differs between the various types of AI, offering some practical examples.
Continue reading.
Table of contents
What is artificial intelligence?
First of all, what is artificial intelligence? AI is the science of creating computer systems capable of performing tasks that normally require human intelligence, such as object recognition, natural language understanding, problem solving and learning.
In other words, AI is a branch of computer science that studies the design of intelligent agents, i.e. systems capable of perceiving their environment and acting in pursuit of specific goals.
AI, therefore, is the ability of machines to simulate human cognitive abilities, such as reasoning, planning and creativity.
AI falls into 3 categories.
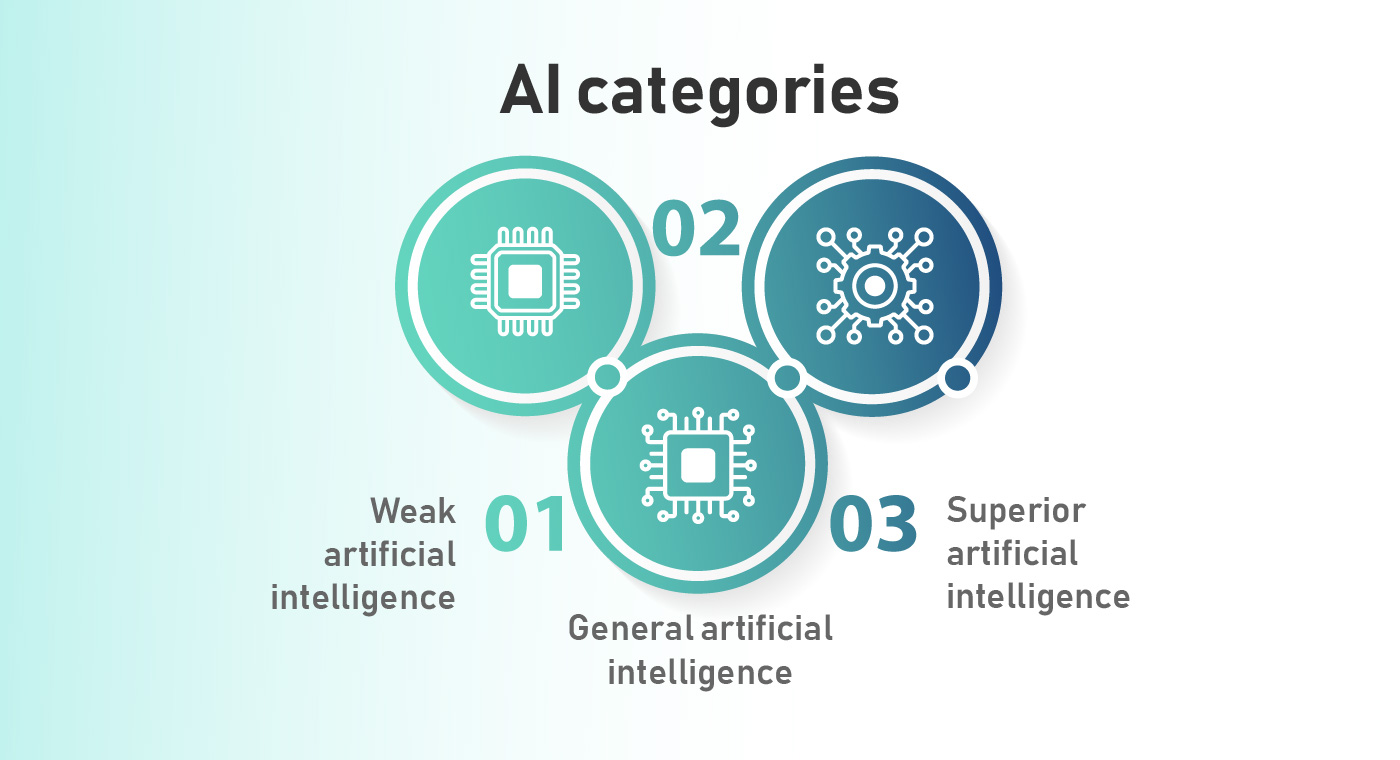
1. Weak artificial intelligence
Weak artificial intelligence refers to those systems that perform only one type of task, such as winning a chess game or identifying a person within a photo. This is the most widespread and realised form of AI today.
2. General artificial intelligence
General artificial intelligence, instead, concerns more complex systems capable of reaching the same level of intelligence as a human being with an average IQ and performing tasks such as understanding context, humour and emotions. This is the most ambitious and challenging form of AI, still under development.2
3. Superior artificial intelligence
A superior artificial intelligence does not exist today; if it did, it would surpass the intelligence of any human being and could perform tasks that are not available to him, such as creating new life forms or colonising other planets. It’s probably the most speculative and controversial form of AI, which could have unpredictable outcomes for mankind.
What is machine learning?
Machine learning is a branch of artificial intelligence concerning the ability of complex systems to learn automatically from clusters of data in order to make predictions or decisions.
Machine learning is based on mathematical algorithms that analyse data, identify patterns and build predictive models.
There are 2 types of ML.
1. Supervised machine learning
Supervised machine learning involves systems learning of labelled data, i.e. data containing both input and output information. For example, a supervised machine learning system can classify e-mails into spam or non-spam, using a set of e-mails already labelled as such.
2. Unsupervised machine learning
Unsupervised machine learning uses, differently, unlabelled data learning systems, i.e. data that do not contain both input and output information, but only the former. In this case, an unsupervised machine learning system knows how to group images according to their similarity, without knowing a priori to which categories they belong.
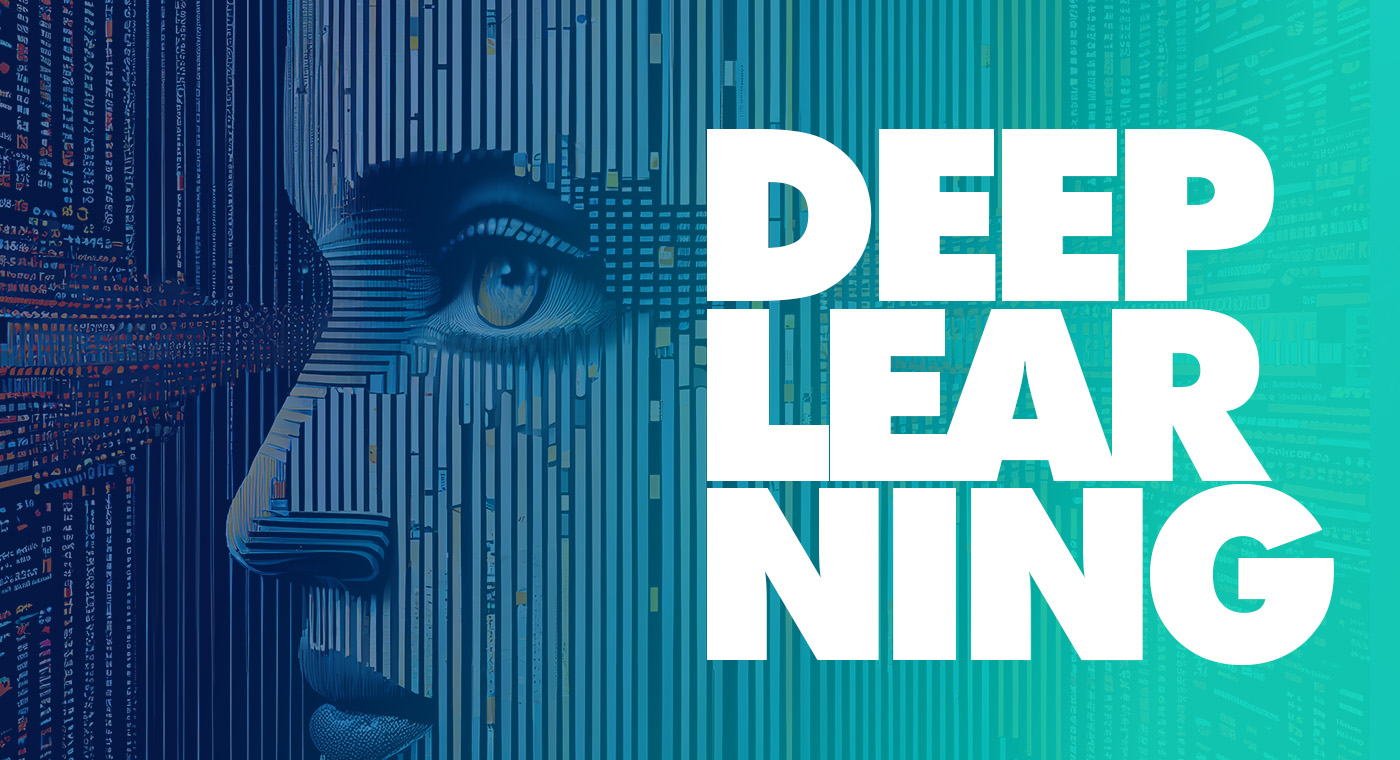
What is deep learning?
Deep learning is a further sub-branch of machine learning; it deals with creating complex artificial intelligence systems based on artificial neural networks.
Artificial neural networks are computational models inspired by the functioning of the human brain, consisting of several layers of units (usually two or three), called neurons. Each neuron receives input from the neurons of the previous layers, processes them using a complex mathematical function and produces an output for the neurons of the next layer, and so on.
Deep learning owes its name to the use of deep neural networks, i.e. with many layers of neurons, capable of learning complex and abstract representations of data that would otherwise be impossible to achieve with traditional machine learning methods.
The success of deep learning today is due to the great availability of big data and, on the hardware side, the advent of powerful central processing units (CPU) and graphics (GPU), which accelerate learning.
The results are surprising and find application in several areas, such as:
- facial recognition;
- machine translation;
- image and text generation;
- medical diagnosis;
- autonomous driving.
What are the differences between deep learning, machine learning and traditional AI?
As we have seen, deep learning, machine learning and traditional AI are related concepts, but with significant differences. We can summarise them as follows:
- traditional AI is based on logical and symbolic rules defined by experts. It’s effective for solving well-defined and structured problems, but has limitations when it comes to handling complex, variable and unstructured data;
- machine learning uses statistical algorithms to learn autonomously from data and thus solve problems requiring adaptation and generalisation, but has limitations when dealing with sparse, noisy or high-dimensional data;
- deep learning is based on artificial neural networks that learn in a hierarchical and non-linear manner. Like machine learning, it’s used to solve complex problems with the difference that, since it involves a deeper and more abstract understanding, it requires large computational resources, with the only limits being data robustness and security.
Deep learning vs machine learning
Deep learning algorithms, compared to those used by machine learning, are more “malleable”, i.e. have a more dynamic code, and are used for analysing large amounts of data.
Moreover, deep learning
- does not require feature design, as neural networks generate the required features automatically and autonomously from raw data;
- can work with unstructured datasets consisting of image, video and audio files (machine learning, on the other hand, works best with tabular data);
- requires large amounts of training data, in the order of thousands or millions of examples;
- has a greater capacity for abstraction and learning of complex concepts;
- it’s computationally more intensive, i.e. it requires more powerful hardware resources.
Deep learning vs traditional AI
Traditional AI, also called symbolic AI, uses predefined rules and formal logic to solve problems. Deep learning, by contrast, learns from experiences (or rather from data, even raw data as we have seen) rather than from rules.
Traditional AI tends to work well in domains that are already defined and limited, but has difficulty handling unexpected situations or scaling large amounts of data. Deep learning, instead, excels in dynamic and complex environments, while requiring huge amounts of data and computing power.
The 5 (possible) best uses of deep learning
According to the multinational Oracle, which sells database software and technologies, the five best possible uses of deep learning are as follows.
Social media
Deep learning can be used to analyse large numbers of images (such as those on social media) to target users.
Finance
Artificial neural networks can predict corporate values, identify threats and/or develop negotiation strategies.
Healthcare
Deep learning can help to understand the behaviour of patients, allowing the best treatment for them to be identified.
IT security
Deep learning algorithms are not only able to detect threats, but also offer effective solutions against viruses, spyware and malware.
Digital assistants
Natural language processing (NLP) allows us to have digital assistants such as Siri, Cortana, Google Assistant, Alexa, etc. that use conversational artificial intelligence to offer us the best answers.
Most important challenges
Although the implementation of deep learning within companies and PA is in the making, there are some important limitations and challenges to be faced, such as:
- the processing of large amounts of data, which requires, as mentioned above, exorbitant hardware resources;
- the lack of flexibility, translated into the inability of machines to act outside their own sphere of competence;
- the lack of transparency, determined by the difficulty of understanding how the AI arrives at the answer.
Despite the aforementioned obstacles, data scientists are getting closer and closer to creating deep learning models that are highly accurate, precise and capable of learning without external supervision.
Deep learning in short
In summary, deep learning creates models that can make autonomous decisions on the basis of examples, without the need for pre-programmed rules. In this sense, deep learning represents a crucial step forward in the development of AI.
It’s the approach that most closely mimics the functioning of the human brain, learning directly from data and its combination, rather than following rigid rules.
A “behaviour”, in conclusion, that allows deep learning to perform better than machine learning and traditional AI, especially in tasks such as voice and image recognition and machine translation.
Choose PMF Research to develop deep learning projects
PMF Reserach is a research and development (R&D) centre established in 2003 and part of the JO Group cluster of companies; it deals with ICT, virtual reality, artificial intelligence and big data.
If you found this article interesting, would like to learn more and, why not, realise an entrepreneurial project involving deep learning, please contact us by filling in the contact form below or by calling +390957225331. A team of experts is here to answer all your questions.